Best Practices for Building Reliable AWS Lambda Functions
13/01/2025
33
Table of Contents
Welcome back to the “Mastering AWS Lambda with Bao” series! The previous episode explored how AWS Lambda connects to the world through AWS Lambda triggers and events. Using S3 and DynamoDB Streams triggers, we demonstrated how Lambda automates workflows by processing events from multiple sources. This example provided a foundation for understanding Lambda’s event-driven architecture.
However, building reliable Lambda functions requires more than understanding how triggers work. To create AWS lambda functions that can handle real-world production workloads, you need to focus on optimizing performance, implementing robust error handling, and enforcing strong security practices. These steps optimize your Lambda functions to be scalable, efficient, and secure.
In this episode, SupremeTech will explore the best practices for building reliable AWS Lambda functions, covering two essential areas:
- Optimizing Performance: Reducing latency, managing resources, and improving runtime efficiency.
- Error Handling and Logging: Capturing meaningful errors, logging effectively with CloudWatch, and setting up retries.
Adopting these best practices, you’ll be well-equipped to optimize Lambda functions that thrive in production environments. Let’s dive in!
Optimizing Performance
Optimize the Lambda function’s performance to run efficiently with minimal latency and cost. Let’s focus first on Cold Starts, a critical area of concern for most developers.
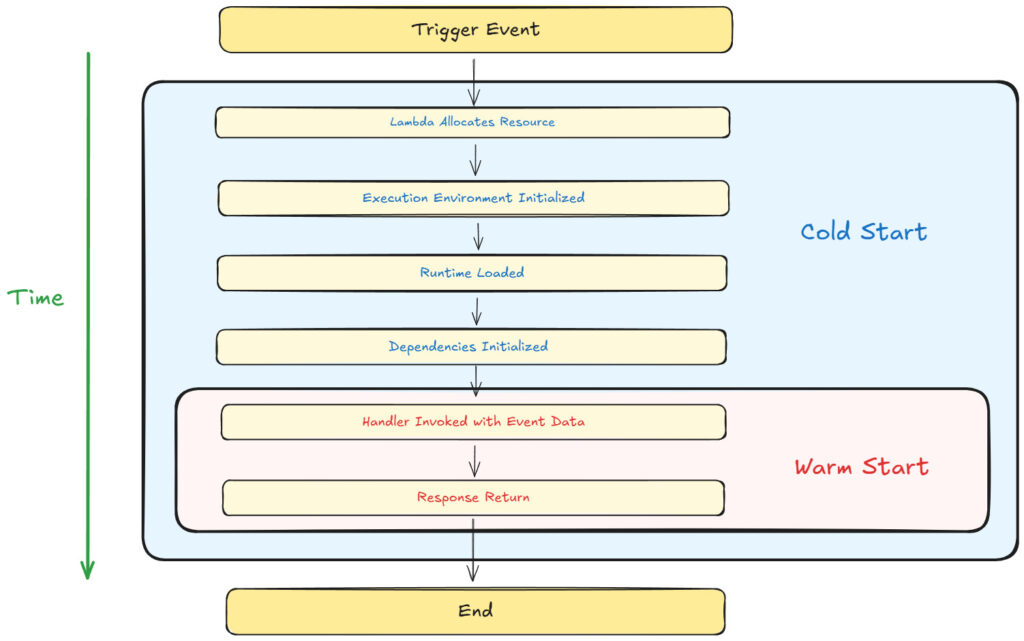
Understanding Cold Starts
What Are Cold Starts?
A Cold Start occurs when AWS Lambda initializes a new execution environment to handle an incoming request. This happens under the following circumstances:
- When the Lambda function is invoked for the first time.
- After a period of inactivity (execution environments are garbage collected after a few minutes of no activity – meaning it will be shut down automatically).
- When scaling up to handle additional concurrent requests.
Cold starts introduce latency because AWS needs to set up a new execution environment from scratch.
Steps Involved in a Cold Start:
- Resource Allocation:
- AWS provisions a secure and isolated container for the Lambda function.
- Resources like memory and CPU are allocated based on the function’s configuration.
- Execution Environment Initialization:
- AWS sets up the sandbox environment, including:
- The
/tmp
directory is for temporary storage. - Networking configurations, such as Elastic Network Interfaces (ENI), for VPC-based Lambdas.
- The
- AWS sets up the sandbox environment, including:
- Runtime Initialization:
- The specified runtime (e.g., Node.js, Python, Java) is initialized.
- For Node.js, this involves loading the JavaScript engine (V8) and runtime APIs.
- Dependency Initialization:
- AWS loads the deployment package (your Lambda code and dependencies).
- Any initialization code in your function (e.g., database connections, library imports) is executed.
- Handler Invocation:
- Once the environment is fully set up, AWS invokes your Lambda function’s handler with the input event.
Cold Start Latency
Cold start latency varies depending on the runtime, deployment package size, and whether the function runs inside a VPC:
- Node.js and Python: ~200ms–500ms for non-VPC functions.
- Java or .NET: ~500ms–2s due to heavier runtime initialization.
- VPC-Based Functions: Add ~500ms–1s due to ENI initialization.
Warm Starts
In contrast to cold starts, Warm Starts reuse an already-initialized execution environment. AWS keeps environments “warm” for a short time after a function is invoked, allowing subsequent requests to bypass initialization steps.
Key Differences:
- Cold Start: New container setup → High latency.
- Warm Start: Reused container → Minimal latency (~<100ms).
Reducing Cold Starts
Cold starts can significantly impact the performance of latency-sensitive applications. Below are some actionable strategies to reduce cold starts, each with good and bad practice examples for clarity.
1. Use Smaller Deployment Packages to optimize lambda function
Good Practice:
- Minimize the size of your deployment package by including only the required dependencies and removing unnecessary files.
- Use bundlers like Webpack, ESBuild, or Parcel to optimize your package size.
- Example:
const DynamoDB = require('aws-sdk/clients/dynamodb'); // Only loads DynamoDB, not the entire SDK
Bad Practice:
- Bundling the entire AWS SDK or other large libraries without considering modular imports.
- Example:
const AWS = require('aws-sdk'); // Loads the entire SDK, increasing package size
Why It Matters: Smaller deployment packages load faster during the initialization phase, reducing cold start latency.
2. Move Heavy Initialization Outside the Handler
Good Practice:
- Place resource-heavy operations, such as database or SDK client initialization, outside the handler function so they are executed only once per container lifecycle – a cold start.
- Example:
const DynamoDB = new AWS.DynamoDB.DocumentClient(); exports.handler = async (event) => { const data = await DynamoDB.get({ Key: { id: '123' } }).promise(); return data; };
Bad Practice:
- Reinitializing resources inside the handler for every invocation.
- Example:
exports.handler = async (event) => { const DynamoDB = new AWS.DynamoDB.DocumentClient(); // Initialized on every call const data = await DynamoDB.get({ Key: { id: '123' } }).promise(); return data; };
Why It Matters: Reinitializing resources for every invocation increases latency and consumes unnecessary computing power.
3. Enable Provisioned Concurrency1
Good Practice:
- Use Provisioned Concurrency to pre-initialize a set number of environments, ensuring they are always ready to handle requests.
- Example:
- AWS CLI:
aws lambda put-provisioned-concurrency-config \ --function-name myFunction \ --provisioned-concurrent-executions 5
- AWS Management Console:
Why It Matters: Provisioned concurrency ensures a constant pool of pre-initialized environments, eliminating cold starts entirely for latency-sensitive applications.
4. Reduce Dependencies to optimize the lambda function
Good Practice:
- Evaluate your libraries and replace heavy frameworks with lightweight alternatives or native APIs.
- Example:
console.log(new Date().toISOString()); // Native JavaScript API
Bad Practice:
- Using heavy libraries for simple tasks without considering alternatives.
- Example:
const moment = require('moment'); console.log(moment().format());
Why It Matters: Large dependencies increase the deployment package size, leading to slower initialization during cold starts.
5. Avoid Unnecessary VPC Configurations
Good Practice:
- Place Lambda functions outside a VPC unless necessary. If a VPC is required (e.g., to access private resources like RDS), optimize networking using VPC endpoints.
- Example:
- Use DynamoDB and S3 directly without placing the Lambda inside a VPC.
Bad Practice:
- Deploying Lambda functions inside a VPC unnecessarily, such as accessing services like DynamoDB or S3, which do not require VPC access.
- Why It’s Bad: Placing Lambda in a VPC introduces additional latency due to ENI setup during cold starts.
Why It Matters: Functions outside a VPC initialize faster because they skip ENI setup.
6. Choose Lightweight Runtimes to optimize lambda function
Good Practice:
- Use lightweight runtimes like Node.js or Python for faster initialization than heavier runtimes like Java or .NET.
- Why It’s Good: Lightweight runtimes require fewer initialization resources, leading to lower cold start latency.
Why It Matters: Heavier runtimes have higher cold start latency due to the complexity of their initialization process.
Summary of Best Practices for Cold Starts
Aspect | Good Practice | Bad Practice |
Deployment Package | Use small packages with only the required dependencies. | Bundle unused libraries, increasing the package size. |
Initialization | Perform heavy initialization (e.g., database connections) outside the handler. | Initialize resources inside the handler for every request. |
Provisioned Concurrency | Enable provisioned concurrency for latency-sensitive applications. | Ignore provisioned concurrency for high-traffic functions. |
Dependencies | Use lightweight libraries or native APIs for simple tasks. | Use heavy libraries like moment.js without evaluating lightweight alternatives. |
VPC Configuration | Avoid unnecessary VPC configurations; use VPC endpoints when required. | Place all Lambda functions inside a VPC, even when accessing public AWS services. |
Runtime Selection | Choose lightweight runtimes like Node.js or Python for faster initialization. | Use heavy runtimes like Java or .NET for simple, lightweight workloads. |
Error Handling and Logging
Error handling and logging are critical for optimizing your Lambda functions are reliable and easy to debug. Effective error handling prevents cascading failures in your architecture, while good logging practices help you monitor and troubleshoot issues efficiently.
Structured Error Responses
Errors in Lambda functions can occur due to various reasons: invalid input, AWS service failures, or unhandled exceptions in the code. Properly structured error handling ensures that these issues are captured, logged, and surfaced effectively to users or downstream services.
1. Define Consistent Error Structures
Good Practice:
- Use a standard error format so all errors are predictable and machine-readable.
- Example:
{ "errorType": "ValidationError", "message": "Invalid input: 'email' is missing", "requestId": "12345-abcd" }
Bad Practice:
- Avoid returning vague or unstructured errors that make debugging difficult.
{ "message": "Something went wrong", "error": true }
Why It Matters: Structured errors make debugging easier by providing consistent, machine-readable information. They also improve communication with clients or downstream systems by conveying what went wrong and how it should be handled.
2. Use Custom Error Classes
Good Practice:
- In Node.js, define custom error classes for clarity:
class ValidationError extends Error { constructor(message) { super(message); this.name = "ValidationError"; this.statusCode = 400; // Custom property } } // Throwing a custom error if (!event.body.email) { throw new ValidationError("Invalid input: 'email' is missing"); }
Bad Practice:
- Use generic errors for everything, making identifying or categorizing issues hard.
- Example:
throw new Error("Error occurred");
Why It Matters: Custom error classes make error handling more precise and help segregate application errors (e.g., validation issues) from system errors (e.g., database failures).
3. Include Contextual Information in Logs
Good Practice:
- Add relevant information like
requestId
,timestamp
, and input data (excluding sensitive information) when logging errors. - Example:
console.error({ errorType: "ValidationError", message: "The 'email' field is missing.", requestId: context.awsRequestId, input: event.body, timestamp: new Date().toISOString(), });
Bad Practice:
- Log errors without any context, making debugging difficult.
- Example:
console.error("Error occurred");
Why It Matters: Contextual information in logs makes it easier to identify what triggered the error and where it happened, improving the debugging experience.
Retry Logic Across AWS SDK and Other Services
Retrying failed operations is critical when interacting with external services, as temporary failures (e.g., throttling, timeouts, or transient network issues) can disrupt workflows. Whether you’re using AWS SDK, third-party APIs, or internal services, applying retry logic effectively can ensure system reliability while avoiding unnecessary overhead.
1. Use Exponential Backoff and Jitter
Good Practice:
- Apply exponential backoff with jitter to stagger retry attempts. This avoids overwhelming the target service, especially under high load or rate-limiting scenarios.
- Example (General Implementation):
async function retryWithBackoff(fn, retries = 3, delay = 100) { for (let attempt = 1; attempt <= retries; attempt++) { try { return await fn(); } catch (error) { if (attempt === retries) throw error; // Rethrow after final attempt const backoff = delay * 2 ** (attempt - 1) + Math.random() * delay; // Add jitter console.log(`Retrying in ${backoff.toFixed()}ms...`); await new Promise((res) => setTimeout(res, backoff)); } } } // Usage Example const result = await retryWithBackoff(() => callThirdPartyAPI());
Bad Practice:
- Retrying without delays or jitter can lead to cascading failures and amplify the problem.
for (let i = 0; i < retries; i++) { try { return await callThirdPartyAPI(); } catch (error) { console.log("Retrying immediately..."); } }
Why It Matters: Exponential backoff reduces pressure on the failing service, while jitter randomizes retry times, preventing synchronized retry storms from multiple clients.
2. Leverage Built-In Retry Mechanisms
Good Practice:
- Use the built-in retry logic of libraries, SDKs, or APIs whenever available. These are typically optimized for the specific service.
- Example (AWS SDK):
const DynamoDB = new AWS.DynamoDB.DocumentClient({ maxRetries: 3, // Number of retries retryDelayOptions: { base: 200 }, // Base delay in ms });
- Example (Axios for Third-Party APIs):
- Use libraries like
axios-retry
to integrate retry logic for HTTP requests.
- Use libraries like
const axios = require('axios'); const axiosRetry = require('axios-retry'); axiosRetry(axios, { retries: 3, // Retry 3 times retryDelay: (retryCount) => retryCount * 200, // Exponential backoff retryCondition: (error) => error.response.status >= 500, // Retry only for server errors }); const response = await axios.get("https://example.com/api");
Bad Practice:
- Writing your own retry logic unnecessarily when built-in mechanisms exist, risking suboptimal implementation.
Why It Matters: Built-in retry mechanisms are often optimized for the specific service or library, reducing the likelihood of bugs and configuration errors.
3. Configure Service-Specific Retry Limits
Good Practice:
- Set retry limits based on the service’s characteristics and criticality.
- Example (AWS S3 Upload):
const s3 = new AWS.S3({ maxRetries: 5, // Allow more retries for critical operations retryDelayOptions: { base: 300 }, // Slightly longer base delay });
- Example (Database Queries):
async function queryDatabaseWithRetry(queryFn) { await retryWithBackoff(queryFn, 5, 100); // Retry with custom backoff logic }
Bad Practice:
- Allowing unlimited retries can cause resource exhaustion and increase costs.
while (true) { try { return await callService(); } catch (error) { console.log("Retrying..."); } }
Why It Matters: Excessive retries can lead to runaway costs or cascading failures across the system. Always define a sensible retry limit.
4. Handle Transient vs. Persistent Failures
Good Practice:
- Retry only transient failures (e.g., timeouts, throttling, 5xx errors) and handle persistent failures (e.g., invalid input, 4xx errors) immediately.
- Example:
const isTransientError = (error) => error.code === "ThrottlingException" || error.code === "TimeoutError"; async function callServiceWithRetry() { await retryWithBackoff(() => { if (!isTransientError(error)) throw error; // Do not retry persistent errors return callService(); }); }
Bad Practice:
- Retrying all errors indiscriminately, including persistent failures like
ValidationException
or404 Not Found
.
Why It Matters: Persistent failures are unlikely to succeed with retries and can waste resources unnecessarily.
5. Log Retry Attempts
Good Practice:
- Log each retry attempt with relevant context, such as the retry count and delay.
async function retryWithBackoff(fn, retries = 3, delay = 100) { for (let attempt = 1; attempt <= retries; attempt++) { try { return await fn(); } catch (error) { if (attempt === retries) throw error; console.log(`Attempt ${attempt} failed. Retrying in ${delay}ms...`); await new Promise((res) => setTimeout(res, delay)); } } }
Bad Practice:
- Failing to log retries makes debugging or understanding the retry behavior difficult.
Why It Matters: Logs provide valuable insights into system behavior and help diagnose retry-related issues.
Summary of Best Practices for Retry logic
Aspect | Good Practice | Bad Practice |
Retry Logic | Use exponential backoff with jitter to stagger retries. | Retry immediately without delays, causing retry storms. |
Built-In Mechanisms | Leverage AWS SDK retry options or third-party libraries like axios-retry . | Write custom retry logic unnecessarily when optimized built-in solutions are available. |
Retry Limits | Define a sensible retry limit (e.g., 3–5 retries). | Allow unlimited retries, risking resource exhaustion or runaway costs. |
Transient vs Persistent | Retry only transient errors (e.g., timeouts, throttling) and fail fast for persistent errors. | Retry all errors indiscriminately, including persistent failures like validation or 404 errors. |
Logging | Log retry attempts with context (e.g., attempt number, delay, error) to aid debugging. | Fail to log retries, making it hard to trace retry behavior or diagnose problems. |
Logging Best Practices
Logs are essential for debugging and monitoring Lambda functions. However, unstructured or excessive logging can make it harder to find helpful information.
1. Mask or Exclude Sensitive Data
Good Practice:
- Avoid logging sensitive information like:
- User credentials
- API keys, tokens, or secrets
- Personally Identifiable Information (PII)
- Use tools like AWS Secrets Manager for sensitive data management.
- Example: Mask sensitive fields before logging:
const sanitizedInput = { ...event, password: "***", }; console.log(JSON.stringify({ level: "info", message: "User login attempt logged.", input: sanitizedInput, }));
Bad Practice:
- Logging sensitive data directly can cause security breaches or compliance violations (e.g., GDPR, HIPAA).
- Example:
console.log(`User logged in with password: ${event.password}`);
Why It Matters: Logging sensitive data can expose systems to attackers, breach compliance rules, and compromise user trust.
2. Set Log Retention Policies
Good Practice:
- Set a retention policy for CloudWatch log groups to prevent excessive log storage costs.
- AWS allows you to configure retention settings (e.g., 7, 14, or 30 days).
Bad Practice:
- Using the default “Never Expire” retention policy unnecessarily stores logs indefinitely.
Why It Matters: Unmanaged logs increase costs and make it harder to find relevant data. Retaining logs only as long as needed reduces costs and keeps logs manageable.
3. Avoid Excessive Logging
Good Practice:
- Log only what is necessary to monitor, troubleshoot, and analyze system behavior.
- Use
info
,debug
, anderror
levels to prioritize logs appropriately.
console.info("Function started processing..."); console.error("Failed to fetch data from DynamoDB: ", error.message);
Bad Practice:
- Logging every detail (e.g., input payloads, execution steps) unnecessarily increases log volume.
- Example:
console.log(`Received event: ${JSON.stringify(event)}`); // Avoid logging full payloads unnecessarily
Why It Matters: Excessive logging clutters log storage, increases costs, and makes it harder to isolate relevant logs.
4. Use Log Levels (Info, Debug, Error)
Good Practice:
- Use different log levels to differentiate between critical and non-critical information.
info
: For general execution logs (e.g., function start, successful completion).debug
: For detailed logs during development or troubleshooting.error
: For failure scenarios requiring immediate attention.
Bad Practice:
- Using a single log level (e.g.,
console.log()
everywhere) without prioritization.
Why It Matters: Log levels make it easier to filter logs based on severity and focus on critical issues in production.
Conclusion
In this episode of “Mastering AWS Lambda with Bao”, we explored critical best practices for building reliable AWS Lambda functions, focusing on optimizing performance, error handling, and logging.
- Optimizing Performance: By reducing cold starts, using smaller deployment packages, lightweight runtimes, and optimizing VPC configurations, you can significantly lower latency and optimize Lambda functions. Strategies like moving initialization outside the handler and leveraging Provisioned Concurrency ensure smoother execution for latency-sensitive applications.
- Error Handling: Implementing structured error responses and custom error classes makes troubleshooting easier and helps differentiate between transient and persistent issues. Handling errors consistently improves system resilience.
- Retry Logic: Applying exponential backoff with jitter, using built-in retry mechanisms, and setting sensible retry limits optimizes that Lambda functions gracefully handle failures without overwhelming dependent services.
- Logging: Effective logging with structured formats, contextual information, log levels, and appropriate retention policies enables better visibility, debugging, and cost control. Avoiding sensitive data in logs ensures security and compliance.
Following these best practices, you can optimize lambda function performance, reduce operational costs, and build scalable, reliable, and secure serverless applications with AWS Lambda.
In the next episode, we’ll dive deeper into “Handling Failures with Dead Letter Queues (DLQs)”, exploring how DLQs act as a safety net for capturing failed events and ensuring no data loss occurs in your workflows. Stay tuned!
Note:
1. Provisioned Concurrency is not a universal solution. While it eliminates cold starts, it also incurs additional costs since pre-initialized environments are billed regardless of usage.
- When to Use:
- Latency-sensitive workloads like APIs or real-time applications where even a slight delay is unacceptable.
- When Not to Use:
- Functions with unpredictable or low invocation rates (e.g., batch jobs, infrequent triggers). For such scenarios, on-demand concurrency may be more cost-effective.
Related Blog